ABSTRACT
Capturing images is a key part of automation for high-level tasks such as scene text recognition. Low-light conditions pose a challenge for high-level perception stacks, which are often optimized on well-lit, artifact-free images. Reconstruction methods for low-light images can produce well-lit counterparts, but typically at the cost of high-frequency details critical for downstream tasks. We propose Diffusion in the Dark (DiD), a diffusion model for low-light image reconstruction for text recognition. DiD provides qualitatively competitive reconstructions with that of state-of-the-art (SOTA), while preserving high-frequency details even in extremely noisy, dark conditions. We demonstrate that DiD, without any task-specific optimization, can outperform SOTA low-light methods in low-light text recognition on real images, bolstering the potential of diffusion models to solve ill-posed inverse problems.
FILES
CITATION
C. Nguyen, E. Chan, A. Bergman, G. Wetzstein, Diffusion in the Dark: A Diffusion Model for Low-Light Text Recognition, WACV 2024
@inproceedings{Nguyen:2024:diffusiondark,
author = {Cindy M. Nguyen and Eric R. Chan and Alexander Bergman and Gordon Wetzstein},
title = {Diffusion in the Dark: A Diffusion Model for Low-Light Text Recognition},
booktitle = {WACV},
year = {2024}
}
Method
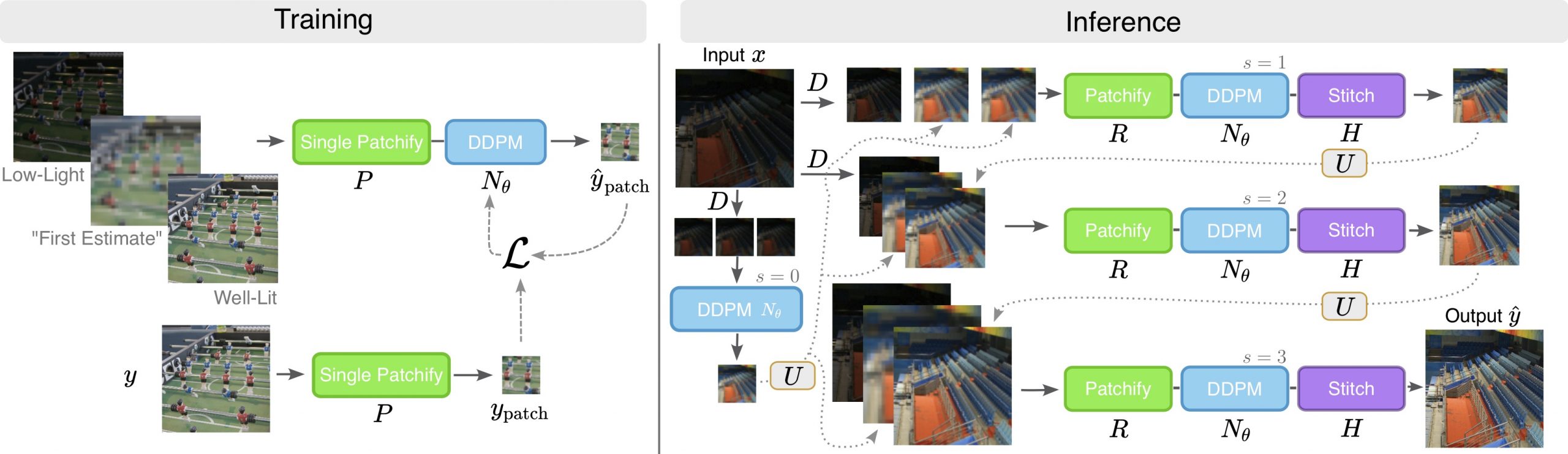 |
Overview of our pipeline. We randomly crop fixed resolution patches at multiple scales and concatenate the low-light patches, low-resolution well-lit patches, and high-resolution well-lit patches together to denoise and reconstruct well-lit patches. This conditioning setup is used in the inference process in which our trained DDPM network is used 4 times successively, each using progressively better resolution well-lit images, to reconstruct well-lit patches. The patches are stitched together to reconstruct the full resolution well-lit image. |